Nvidia Ups the Ante in AI Chip Race with New Blackwell Architecture
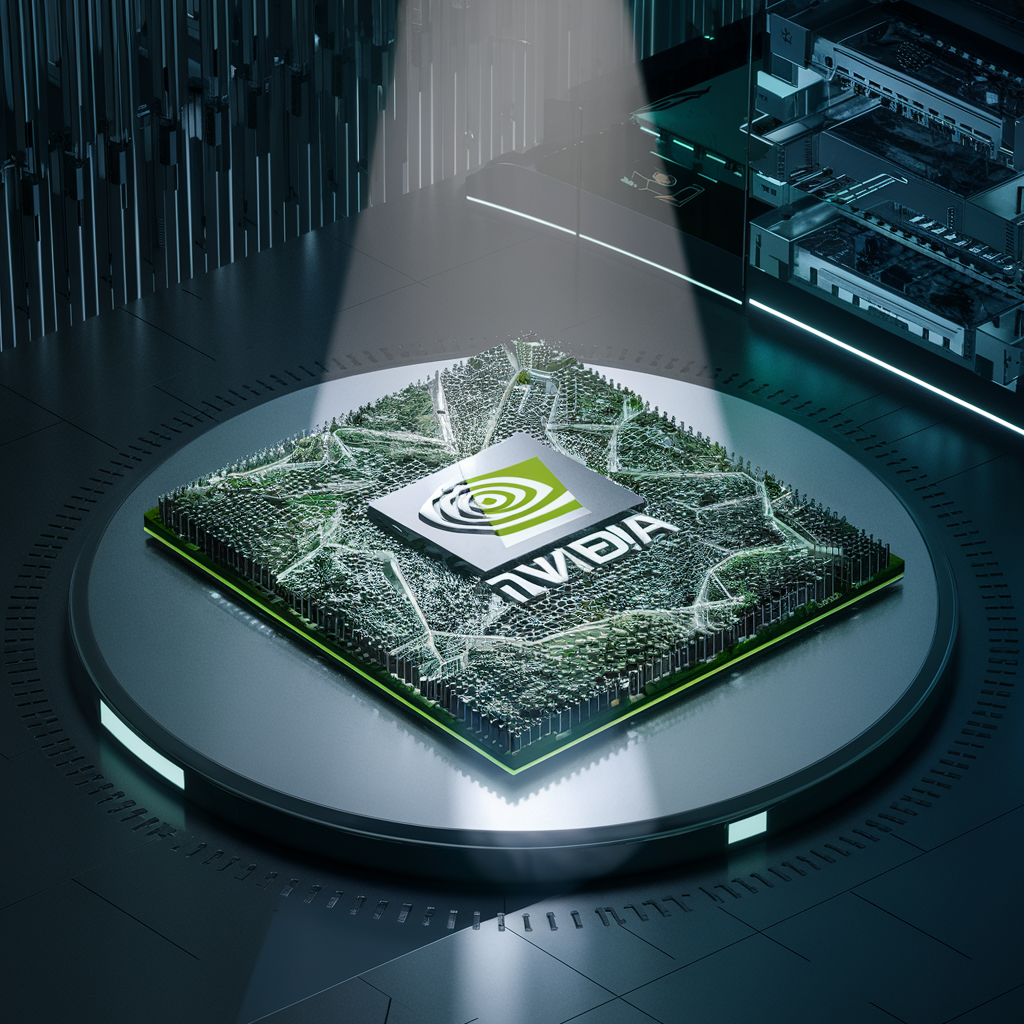
Nvidia is stepping up its game in the artificial intelligence chip market with the announcement of its Blackwell GPU architecture at its first in-person GPU Technology Conference (GTC) in five years.
The new chip, tailored for large data centers such as those powering AWS, Azure, and Google, boasts 20 PetaFLOPS of AI performance. Nvidia claims it is four times faster on AI-training workloads, 30 times faster on AI-inferencing workloads, and up to 25 times more power-efficient than its predecessor.
Compared to the previous H100 “Hopper,” the B200 Blackwell is not only more powerful but also more energy-efficient. Training an AI model the size of GPT-4, which requires 8,000 H100 chips and 15 megawatts of power, would only need 2,000 B200 chips and four megawatts.
“This marks the first significant advance in chip design since the Hopper architecture debuted two years ago,” commented Bob O’Donnell, founder and chief analyst of Technalysis Research, in his LinkedIn newsletter.
Repackaging or Innovation?
Sebastien Jean, CTO of Phison Electronics, a Taiwanese company, described the chip as a “repackaging exercise.”
“It’s beneficial but not groundbreaking,” he told TechNewsWorld. “It’s faster, uses less power, and allows more compute in a smaller area, but fundamentally, it’s the same technology compressed into a smaller form factor.”
Jean pointed out that this makes the results easily replicable by competitors. However, Nvidia’s strategy of being first allows them to maintain a lead while competitors play catch-up.
“Being aggressive and first to market allows Nvidia to establish itself as the sole innovator, driving demand for its products,” he explained. Though Blackwell may seem like a repackaging exercise, it has practical benefits. “In real terms, users will be able to compute more, faster, within the same power and space constraints.”
Significant Advancements
O’Donnell highlighted the Blackwell architecture’s second-generation transformer engine, which reduces AI floating point calculations to four bits from eight bits. “This change alone can double the compute performance and support larger model sizes,” he noted.
The new chips are also backward-compatible with previous versions. “If you have Nvidia systems with H100, Blackwell is plug-compatible,” said Jack E. Gold, founder and principal analyst at J.Gold Associates. However, the high cost of these chips — $30,000 to $40,000 each for the H100 — might be a barrier for some.
Gold added that Blackwell could help developers enhance AI applications by allowing the analysis of more data points, thus improving AI performance.
Nvidia also introduced Nvidia Inference Microservices (NIM) at the GTC. “NIM tools, built on Nvidia’s CUDA platform, will help businesses bring custom applications and pretrained AI models into production,” noted Brian Colello, an equity strategist at Morningstar Research Services.
Supporting AI Deployment
“Large companies with data centers can quickly adopt and deploy new technologies, but small and medium businesses often lack the resources to do so. NIM can help these smaller businesses adopt new technologies more easily,” explained Shane Rau, a semiconductor analyst with IDC.
“With NIM, you can find models specific to your needs, making AI adoption more relevant to individual companies,” he added.
O’Donnell emphasized that while NIM might not be as exciting as the latest hardware, it is crucial for enabling companies to transition from AI experiments to real-world applications. NIM could also create a new revenue stream for Nvidia by allowing licensing on a per GPU/per hour basis.
Nvidia’s Dominance
Rau predicted that Nvidia will continue to dominate the AI processing market, although competitors like AMD and Intel might capture modest shares. Different chips such as microprocessors, FPGAs, and ASICs will also compete for market share.
“Nvidia faces few threats in this market,” said Abdullah Anwer Ahmed, founder of Serene Data Ops. “Their superior hardware and the CUDA software foundation have been integral to AI advancements for over a decade.”
Ahmed noted that while companies like Amazon, Google, and Microsoft/OpenAI are developing their own AI-optimized chips, these efforts are limited to the largest firms. “Most of the AI industry will continue to rely on Nvidia GPUs.”